Poster – Paper 589
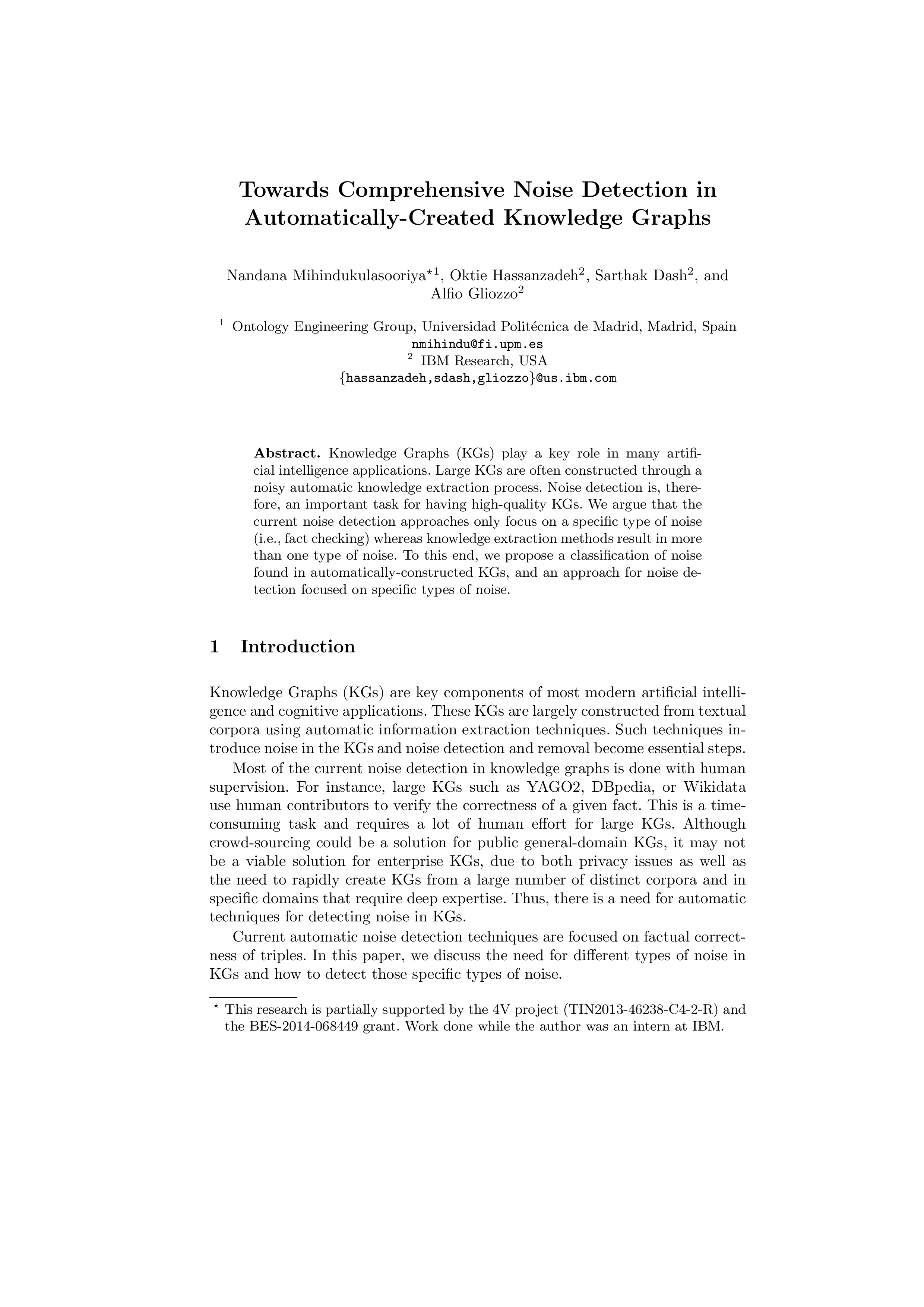 | Nandana Mihindukulasooriya, Oktie Hassanzadeh, Sarthak Dash and Alfio GliozzoPoster
October 23, 2017, Poster and Demo Reception, 18:30-21:20
Festsaal 1
Download paper (preprint) |
Abstract
Knowledge Graphs (KGs) play a key role in many artificial intelligence applications. Large KGs are often constructed through a noisy automatic knowledge extraction process. Noise detection is, therefore, an important task for having high-quality KGs. We argue that the current noise detection approaches only focus on a specific type of noise (i.e., fact checking) whereas knowledge extraction methods result in more than one type of noise. To this end, we propose a classification of noise found in automatically-constructed KGs, and an approach for noise detection focused on specific types of noise.
Leave a Reply (Click here to read the code of conduct)